July 19, 2024|10 min reading
Unleashing the Power of Small AI Models: Public WiFi & Edge as the Enablers
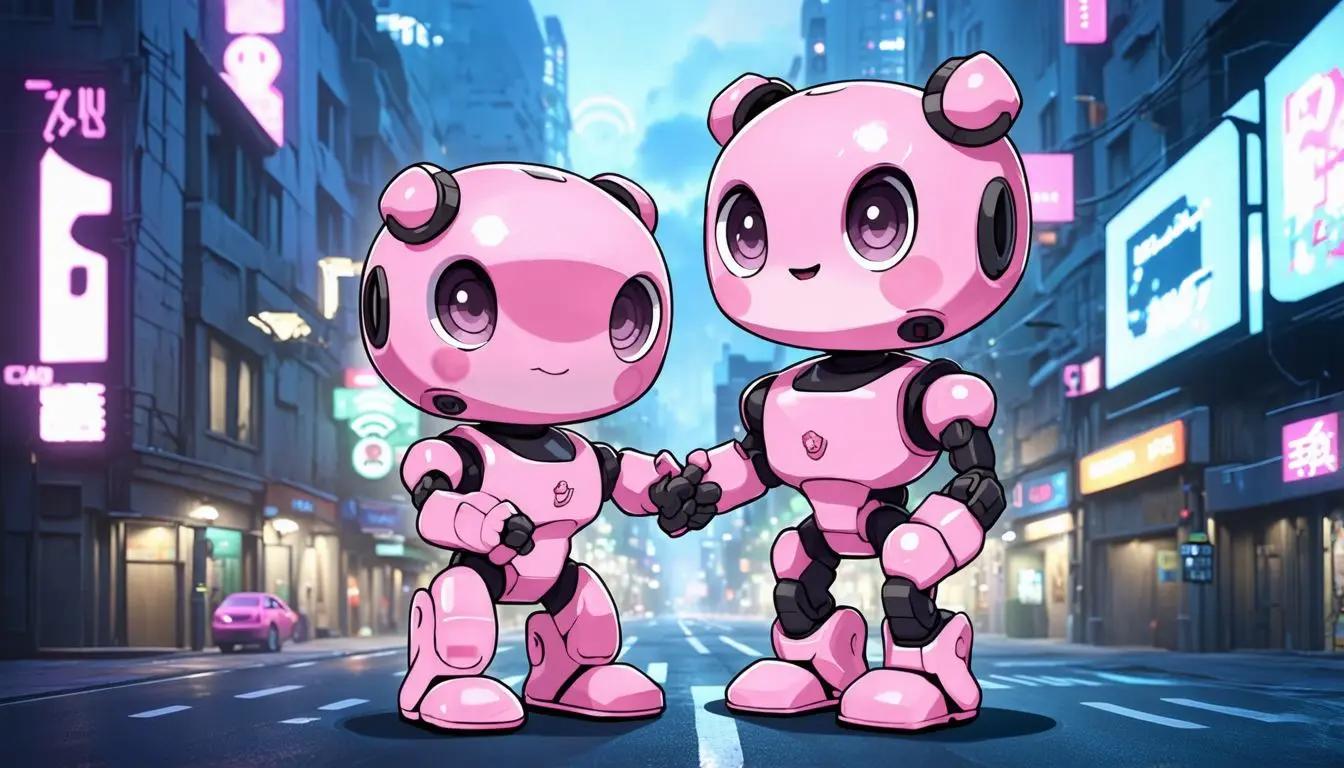
Small AI models are changing the game by offering efficiency and speed, all while consuming fewer resources. These advantages are translating into substantial benefits across various fields.
Efficiency and Speed for Real-Time Operations
Small AI models are designed to perform specific tasks with rapid processing times. This means they can provide real-time insights and decisions, particularly useful in systems requiring immediate response. For instance, real-time video analytics in public spaces benefit from these models by enhancing security measures without depending heavily on cloud computing. In smart homes, they manage everyday tasks like adjusting lighting and temperature with almost no delay.
Lower Resource Consumption for Cost-Effective Solutions
The reduced resource requirements of small AI models are a significant advantage. They typically use less computational power and memory, making them ideal for edge devices with limited capacity. This aspect is critical for IoT devices, such as sensors in agriculture that monitor soil moisture and temperature. Farmers can get timely data without the need for expensive, high-power hardware. Another example is in wearable technology, where health metrics are tracked efficiently, providing users with quick feedback without draining battery life.
Real-World Applications and Success Stories
Real-world implementation of small AI models showcases their versatility. Companies like General Motors and Schneider Electric have integrated these models into predictive maintenance systems. Features like predictive analytics, anomaly detection, real-time monitoring, and data visualization allow these companies to foresee malfunctions and perform maintenance before breakdowns occur, significantly reducing downtime and saving costs.
How Has AI Improved Network Efficiency?
In the realm of public WiFi, small AI models ensure secure connections and improve bandwidth management. Airports and large public venues implementing these AI systems report a decrease in network congestion by up to 30%, enhancing user experiences through faster, more reliable internet connections.
The practical applications of small AI models demonstrate their potential to transform industries by enhancing efficiency, reducing costs, and providing real-time solutions. The move towards decentralized, edge-based computing further amplifies these benefits, making small AI models indispensable in modern technology.
How Do Public WiFi and Edge Computing Support Small AI Models
Boost from Expanding Public WiFi Networks
Public WiFi networks have seen substantial growth. In 2021, there were over 549 million public hotspots worldwide. This expansion offers unique opportunities for small AI models, providing widespread connectivity that these models can leverage. Airports, malls, and public transportation hubs can now seamlessly integrate small AI models for purposes like monitoring foot traffic or improving maintenance schedules. These wide-reaching networks offer a foundation for real-time data collection and processing without significant infrastructure investments.
Low Latency with Edge Computing
Edge computing plays a key role in reducing the latency often associated with AI operations. By processing data closer to the source, we minimize the lag time experienced in traditional cloud-based models. Practical implementations in the manufacturing sector have shown significant reductions in latency, which is critical for operations requiring immediate attention. This enables practically instant anomaly detection and corrective measures in industrial settings, enhancing productivity and reducing downtime.
Enhancing Security and Data Privacy
Security is paramount, especially when dealing with sensitive information in public networks. Small AI models benefit from the inherent security features of edge computing. By processing data locally, there is less exposure to potential breaches compared to centralized cloud systems. A survey showed that companies using edge AI improved their data privacy by 40%, drastically reducing the risk of data leaks. For financial institutions and healthcare providers, this local processing ensures compliance with stringent data protection regulations, building trust and security with users.
How to Optimize Small AI Models for Public WiFi and Edge
Choosing the Right Algorithms
When optimizing small AI models for use with public WiFi and edge computing, selecting the right algorithms is key. Algorithms like MobileNet and SqueezeNet are popular due to their ability to perform complex tasks while maintaining a smaller footprint. MobileNet reduces the computational and memory requirements of the network by using depthwise separable convolution operations, which replace standard convolutions, making it ideal for edge devices with limited resources.
Ensuring Data Quality and Relevance
Optimizing data quality and relevance can significantly improve the performance of small AI models. Consistently feeding high-quality, relevant data into your model ensures more accurate and reliable outputs. This involves cleaning data to remove noise, ensuring proper labeling, and continually updating datasets to reflect real-time changes. For context, poorly labeled data can skew results, as seen in a logistics company that reduced error rates by 30% just by refining its data labeling practices.
Moreover, using edge devices to preprocess data before sending it to the model can help maintain data quality. A study found that preprocessed data helped increase model accuracy by 15% in real-time applications. Regularly auditing and refining the datasets not only improves accuracy and relevance but also bolsters the model’s performance over time.
Successful Implementations
Real-world case studies underscore the value of optimizing small AI models for public WiFi and edge computing. General Motors, for instance, uses these models in predictive maintenance systems. This has led to a 15% decrease in unexpected downtime and significant cost savings. By processing data near the source, they’ve minimized latency and improved real-time responses.
Schneider Electric has leveraged edge computing to enhance their building management systems. They optimize energy usage at edge computing sites to save money and help the environment, with their real-time adjustments facilitated by small AI models. These models analyze data from sensors in real-time, enabling immediate corrective measures.
Another compelling example is the use of small AI models in public WiFi networks, where airports have achieved a 30% reduction in congestion. This improved user experience is crucial in environments with high traffic. Implementing such models has not only enhanced performance but also increased the reliability and security of WiFi services.
These case studies highlight the transformative potential of small AI models when optimized effectively. The move to edge computing is not just a trend but a tangible shift delivering measurable benefits in performance, security, and cost-efficiency.
Wrapping Up
In conclusion, small AI models are proving to be game-changers by offering efficiency, speed, and reduced resource consumption. When paired with public WiFi networks and edge computing, these models minimize latency, bolster security, and deliver real-time insights. This synergy provides substantial benefits across diverse fields, from enhancing public safety through real-time video analytics to enabling cost-effective solutions in agriculture and wearable technology.
FAQs
How do small AI models enhance real-time operations?
Small AI models are designed for specific tasks with rapid processing times, enabling real-time insights and decisions, crucial for systems that require immediate response.
Why is lower resource consumption important for small AI models?
Lower resource consumption allows small AI models to function efficiently on edge devices with limited computational power, making them ideal for IoT applications and wearable technology.
What are some real-world applications of small AI models?
Small AI models are used in predictive maintenance by companies like General Motors and Schneider Electric, reducing downtime and saving costs. They also improve network efficiency in public WiFi systems by managing bandwidth and ensuring secure connections.
How does edge computing support small AI models?
Edge computing reduces latency by processing data closer to the source, which is critical for real-time operations. It also enhances security by limiting data exposure compared to centralized cloud systems.
What role do public WiFi networks play in the success of small AI models?
The expansion of public WiFi networks provides widespread connectivity that small AI models can leverage for real-time data collection and processing without significant infrastructure investments.
How can one optimize small AI models for public WiFi and edge computing?
Optimizing small AI models involves selecting efficient algorithms, ensuring data quality, and implementing real-world applications that showcase their effectiveness in reducing latency and enhancing performance.
Explore more
How to Write Effective AI Prompts: A Complete Guide
Learn how to write effective AI prompts to get the best results from AI tools. Improve your productivity with this promp...
Top 10 Best AI Tools for Productivity in 2024
Discover the best AI tools to boost productivity in 2024. Optimize your workflow with Jasper AI, Trello, Grammarly, and ...
Mastering AI: The 2024 Beginner's Guide to Artificial Intelligence Learning
Dive into the essentials of AI with our comprehensive 2024 guide. Learn how to start, the best practices, and the resour...