June 26, 2024|7 min reading
AI and Programming: A Collaborative Future
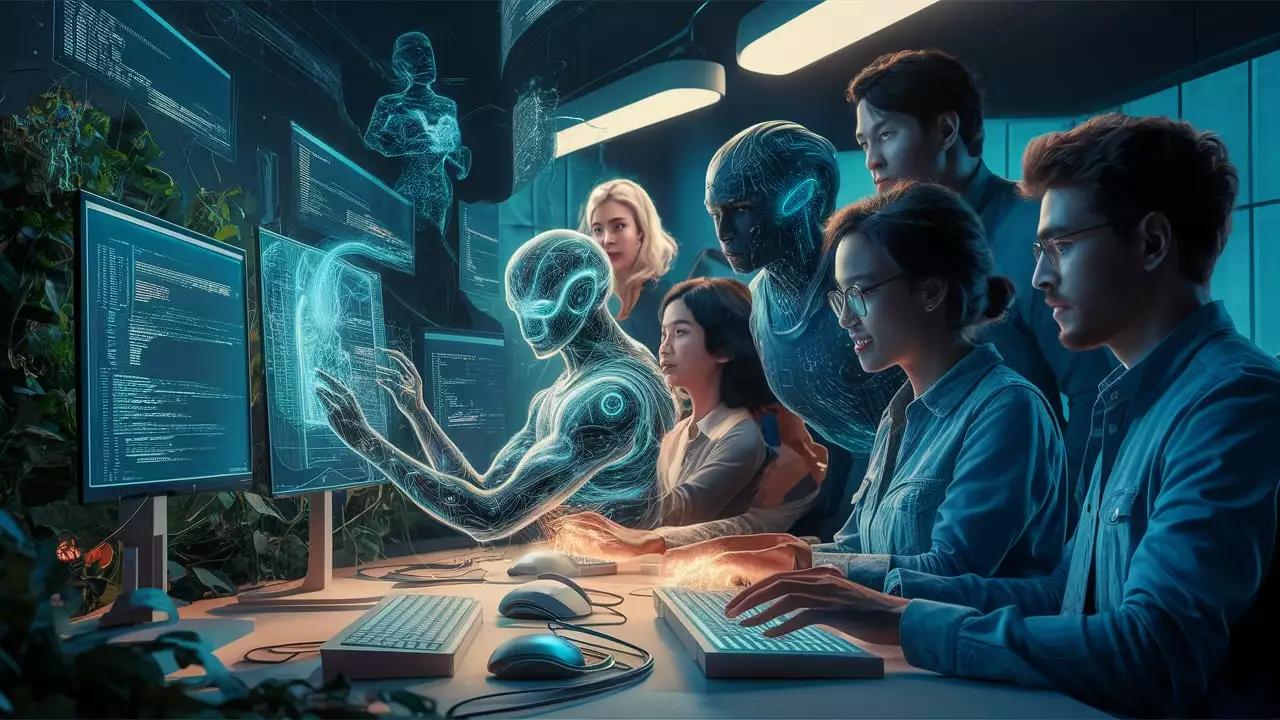
In an era dominated by artificial intelligence (AI), one of the pressing questions is distinguishing between content created by humans and that generated by AI. AI detectors are digital tools designed to address this challenge. These detectors analyze text for patterns, formatting, and other features that might indicate its origin. The primary objective is to determine whether a piece of content was written by a human or an AI.
The Role of AI Detectors in Identifying AI-Generated Content
AI detectors are essential tools for identifying content generated by AI models. They evaluate writing patterns, formatting, and other textual elements to provide a probability score indicating the likelihood of AI authorship. These detectors are crucial in various fields, ensuring authenticity and maintaining trust in digital content.
Types of AI Detectors
AI detectors come in different forms, each with its unique approach:
Perplexity Analysis: This method evaluates the unpredictability in text to identify AI-generated content.
Semantic Coherence: This approach assesses the logical flow and consistency in the text.
Repetitive Patterns: AI content often exhibits repetitive structures, which can be a telltale sign.
Linguistic Fingerprints: Some detectors analyze linguistic features to identify the 'fingerprint' left by AI training data.
These diverse approaches reflect an ongoing technological arms race, as AI evolves to produce more human-like content, and detectors strive to keep up.
Applications of AI Detectors Across Different Fields
AI detectors have significant implications across various sectors:
- Academia: They serve as plagiarism checkers, ensuring the originality of scholarly work.
- Media: Used for content moderation, these tools help identify and filter AI-generated content.
- Finance: AI detectors assist in fraud prevention by detecting AI-generated phishing emails and forged documents.
In all these cases, the primary goal is to maintain authenticity and trust, which AI-generated content might otherwise compromise.
Factors Influencing the Accuracy of AI Detectors
Training Data Quality and Model Complexity
The effectiveness of AI detectors largely depends on the quality of their training data and the complexity of their models. High-quality, diverse training data allows detectors to better differentiate between human and AI-generated text. Complex models, though more accurate, may pose challenges in interpretability, crucial for refining and trusting the system.
Challenges in Distinguishing AI from Human Content
AI-generated content is becoming increasingly sophisticated, making detection more challenging. Detectors need to continuously evolve to keep pace with advancements in AI language models. This rapid evolution can lead to inconsistencies, where newer AI content might evade older detection algorithms.
Bias and Contextual Challenges
Bias in training data can affect the performance of AI detectors, leading to false positives or negatives. Detectors must navigate stylistic variations and contextual nuances to make accurate judgments. For example, a factual text written in an AI-mimicking style might be wrongly flagged, and vice versa.
Case Studies on AI Detector Accuracy
Studies show varying accuracy rates for different AI detectors. For instance:
- One tool reported a 60% success rate in identifying AI-generated text, indicating a considerable margin of error.
- GPTRadar demonstrated a 64% confidence level, highlighting the need for improvement.
- ZeroGPT claimed over 98% accuracy, though such high rates often come with caveats like limited testing conditions.
These studies underscore the need for continuous refinement of AI detection tools to enhance accuracy and reliability.
Ethical Implications of AI Detector Inaccuracy
Ethical Concerns of Misattribution
Inaccurate AI detectors can undermine the integrity of authors by falsely flagging human-created content as AI-generated. This can cause reputational damage and raise questions about authenticity.
Consequences of False Positives and Negatives
False positives can lead to unwarranted scrutiny, while false negatives may allow AI-generated content to pass as authentic. These misclassifications can affect academic integrity, intellectual property rights, and public trust in information authenticity.
Improving the Accuracy of AI Detectors
Addressing Bias and Enhancing Fairness
To mitigate bias, it's crucial to train AI detectors on diverse, representative datasets. Transparency about the limitations and accuracy rates of these tools can help manage expectations.
Continuous Learning and Feedback Mechanisms
AI detectors must continuously evolve by incorporating new data and user feedback. Adaptive learning helps maintain relevancy and accuracy in a rapidly changing digital landscape.
Integrating Human Oversight and Interpretability
Human oversight is essential for navigating the nuances where AI may falter. Transparency in the AI detector's reasoning process enhances trust and allows for better decision-making.
Transparency, Accountability, and Validation Processes
For AI detectors to be reliable, their operations must be transparent, and they should undergo rigorous validation across different datasets and scenarios. Continuous validation ensures that these tools remain effective against evolving AI capabilities.
Conclusion and Future Directions
The future of AI detection lies in robust research and continuous technological advancements. As AI-generated content becomes more sophisticated, detectors must evolve to maintain high accuracy and uphold ethical standards. The integration of human oversight and interpretability is crucial for navigating nuanced cases. By fostering transparency and accountability, we can collectively contribute to the refinement of AI detectors, ensuring they remain reliable and trustworthy tools in the digital age.
Explore more
Black Forest Labs Launches API for Faster Image Generation with Flux1.1 Pro Model
Black Forest Labs unveils its Flux image generator API, providing developers fast, high-quality image generation options...
WebFill: The Ultimate AI Tool for Form Filling, Surveys, and Data Entry
Explore WebFill, an AI-driven tool for automated form filling, survey completion, and data entry. Discover its powerful ...
Meta AI game plan and future trends in AI technology
Discover Meta's AI game plan, future AI trends, and the tools for creators, including the neural wristband and holograph...